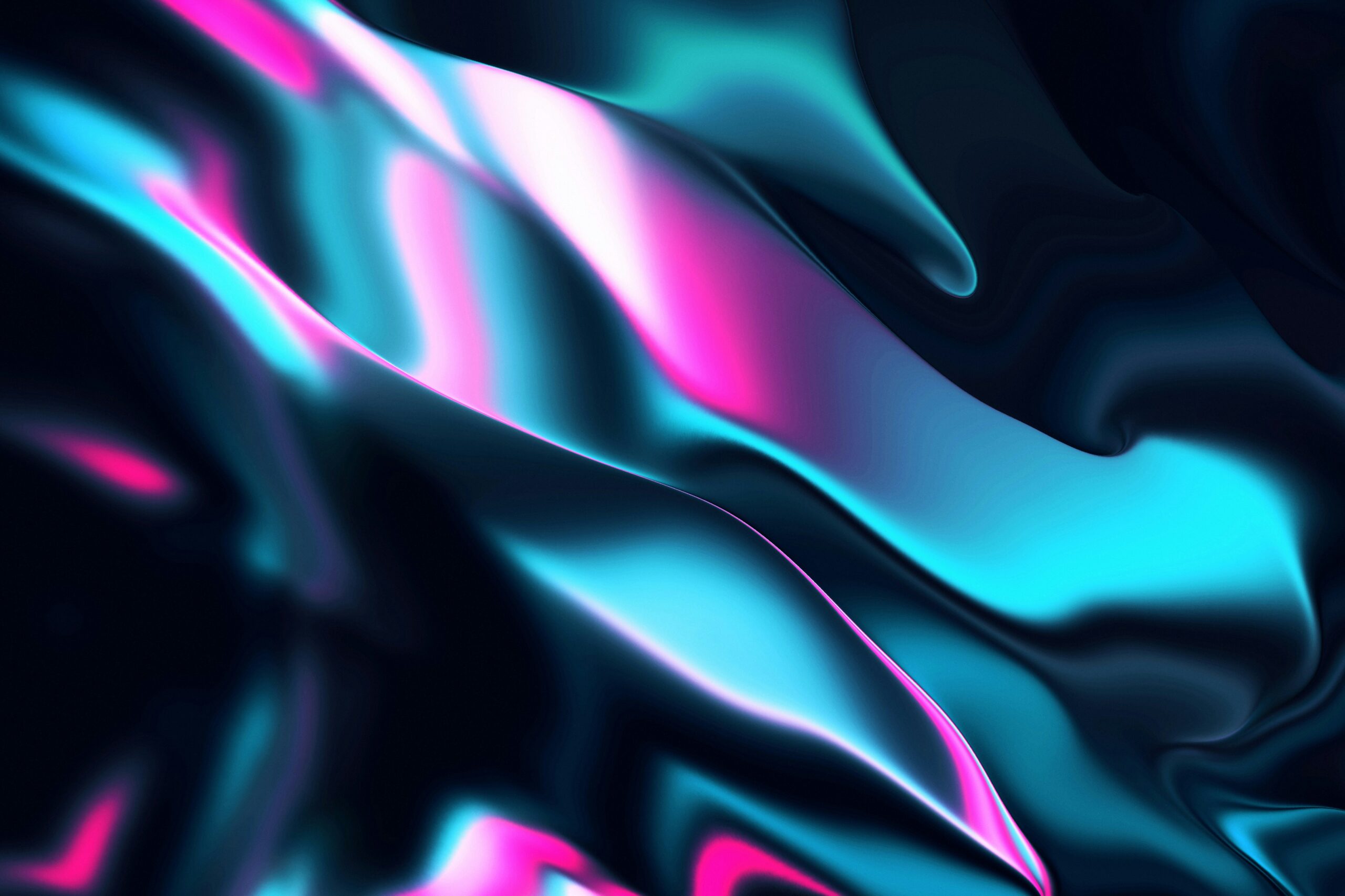
- Dec 15, 2023
- 5 minutes
The landscape of AI has significantly developed and changed over the past year, marking a clear trajectory towards generative models. ChatGPT and Large Language Models (LLMs) in general are currently leading this frontier with promising potential in creating content, generating models, and automating various tasks. It enables machines to produce outputs such as texts, images, or audio that resemble human-generated content.
Yet, as much as these generative tools are fascinating in their existence, they hold even more significant potential for enterprises
Patterns and Use Cases of Generative AI in Enterprises
Generative AI can be applied in different patterns within an enterprise environment. One common application is narrow automation, where AI systems are designed to improve specific tasks or processes, reducing human effort and increasing efficiency. For example, generative AI can significantly improve Information Retrieval from unstructured data, allowing organisation employees to efficiently navigate and extract value of – up until now – unutilized enterprise data.
Another pattern is workflow automation, where generative AI solutions are integrated into existing workflows and systems. This integration enables seamless collaboration between humans and AI, improving productivity and reducing errors. Private instances or dedicated cloud resources of generative AI models can also be deployed within organisations to ensure data privacy and security.
The use of tailored language models (LLMs) is another pattern gaining traction in the enterprise. By training AI models on specific industry or company-specific datasets, LLMs can generate content that aligns with domain-specific requirements. This customisation allows organisations to generate accurate reports, summaries, and recommendations.

Real-world Generative AI Deployments
Adopting generative AI into enterprises is a step-by-step process, each with its unique set of challenges and rewards. More often than not, the journey begins with internal use, wherein the organization learns to adapt to the nuances of generative AI. This transition phase serves as a stepping stone towards applying these technologies to more sensitive data with private instances and tailor-made LLMs.
Organizations often begin by utilising generative AI for internal tasks and processes, such as automating data preparation, report generation, or content creation. These initial use cases help organisations understand the potential benefits and limitations of generative AI within their specific contexts.
As organisations develop confidence and experience, they start incorporating generative AI into more sensitive areas, such as, for instance, contract validation in the banking and insurance industry. Automating the validation process using generative AI can significantly reduce manual effort and improve accuracy.
In the medical field, generative AI finds applications in analysing medical images, aiding in accurate diagnosis, and generating personalised treatment plans. The chemical industry leverages generative AI to optimize chemical reactions and identify novel compounds with desired properties. These use cases highlight the potential of generative AI in transforming industries and driving innovation.
It is crucial to highlight that in all the cases we have seen, processes are not completely automated. Domain experts and professionals always inspect, validate and, if necessary, correct the output of generative AI models, especially when this output is used to either feed other systems or make decisions. This concept is known as human-in-the-loop.
Throughout this journey, organizations refine their AI models, fine-tune their training data, and incorporate user feedback to improve the performance and reliability of generative AI systems. It is often an iterative process involving continuous learning and adaptation.

Common Pitfalls and Solutions in Implementing Generative AI
Implementing generative AI in an enterprise environment can have its challenges. It’s important to be aware of common pitfalls and have solutions in place to overcome them.
One potential pitfall is the risk of information leakage. Generative AI models trained on sensitive data may inadvertently generate outputs that reveal confidential information. To mitigate this risk, organizations must develop robust privacy and security measures, ensuring that generated content does not violate data protection regulations.
Hallucination is another challenge faced in generative AI. AI models may generate content that appears realistic but is factually incorrect or misleading. To address this issue, organizations need to implement rigorous validation processes and practices to ensure the accuracy and reliability of generated outputs.
Neglecting data engineering is a common pitfall in implementing generative AI. High-quality and well-prepared data is crucial for the success of generative AI projects. Organizations must invest in proper data preprocessing, cleaning, and enrichment techniques to deliver reliable and robust AI models.
Furthermore, projects without clear objectives or targets can lead to wasted resources and ineffective output. It is essential to define specific goals and desired outcomes before embarking on generative AI initiatives. This clarity allows organizations to measure the success of their projects and make necessary adjustments along the way.

The Role of Accelerator in Generative AI Projects
One promising way to navigate through the challenges and ensure a more seamless adoption of generative AI in enterprises is by using an Accelerator. It essentially automates common infrastructure tasks in AI projects, ensuring higher security and faster project delivery. With an Accelerator, organizations can leverage the power and potential of generative AI solutions without getting entangled in the complexities and potential risks of the adoption process.
Moreover, Accelerator provides essential security measures to protect sensitive data and mitigate vulnerabilities. It ensures that generative AI systems are built with robust privacy and compliance controls, adhering to industry regulations.
Conclusion
In conclusion, generative AI technologies, such as ChatGPT and LLMs, hold significant potential for shaping the future of enterprises. However, it is crucial to understand the journey of adoption, identify mature use cases, and avoid common pitfalls to fully realize their potential. With the right structure, approaches, and tools like a GenAI Accelerator, an AI-empowered future is within reach.