- Jun 21, 2022
- Walid Mehanna
- Tanvi Singh
- Dr. Luca Baldassarre
- Dr. Marcin Pietrzyk
- Michal Rachtan
Analytics is one of the hottest areas in tech these days. At Unit8, we follow the latest developments in this domain, from academic research to industry innovation. Read more
With the participation of:
On-demand resource
Download the report
Introduction
Analytics is one of the hottest areas in tech these days. At Unit8, we follow the latest developments in this domain, from academic research to industry innovation. As trusted advisors in the analytics domain and based on our expertise and experience working with dozens of industry-leading clients, we have identified four key analytics trends that we will see accelerating in 2022. We recently discussed these trends with analytics experts from Credit Suisse, Merck, and Swiss Re and have distilled this discussion in the report below. I hope you find our predictions insightful and relevant.
Mega models (e.g. GPT-3, Wu Dao 2.0, etc.) show impressive performance yet are extremely costly to train. Only a few companies are able to compete in this space, nonetheless, the availability of these mega models opens the possibilities to new applications. There is still a major challenge around quality control before these are broadly adopted in a business environment but they already assist developers in writing snippets of code.
On the other side, recent developments in ML with semi-supervised learning or synthetic data allow the development of more AI projects, especially in domains where quality labeled data is scarce. These approaches are cost-efficient and prove valuable across industries. They provide a welcomed alternative to the data-intensive approaches from only a few years ago.
Regarding data infrastructures, the young data mesh trend evolved from the data lakes to work as a series of distributed data domains built with common architectural patterns and accessible via understandable, external interfaces. It is powerful but its complexity might not fit companies of all sizes.
Finally, the success of MLOps stems from the need to create a robust process to deploy ML models in production. Moreover, it generates transparency, which is crucial to understand models and incorporate them into the business. MLOps will be a key pillar when it comes to delivering long-term value at scale.
Speakers:
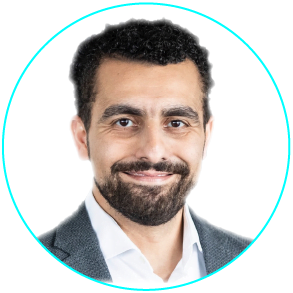
Walid MehannaGroup Data Officer,
Merck Group
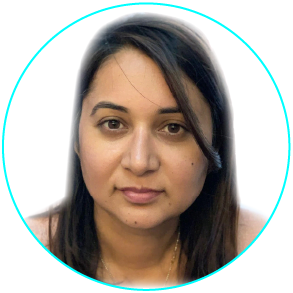
Tanvi SinghCCRO Head of Analytics
and Data Science,
Credit Suisse
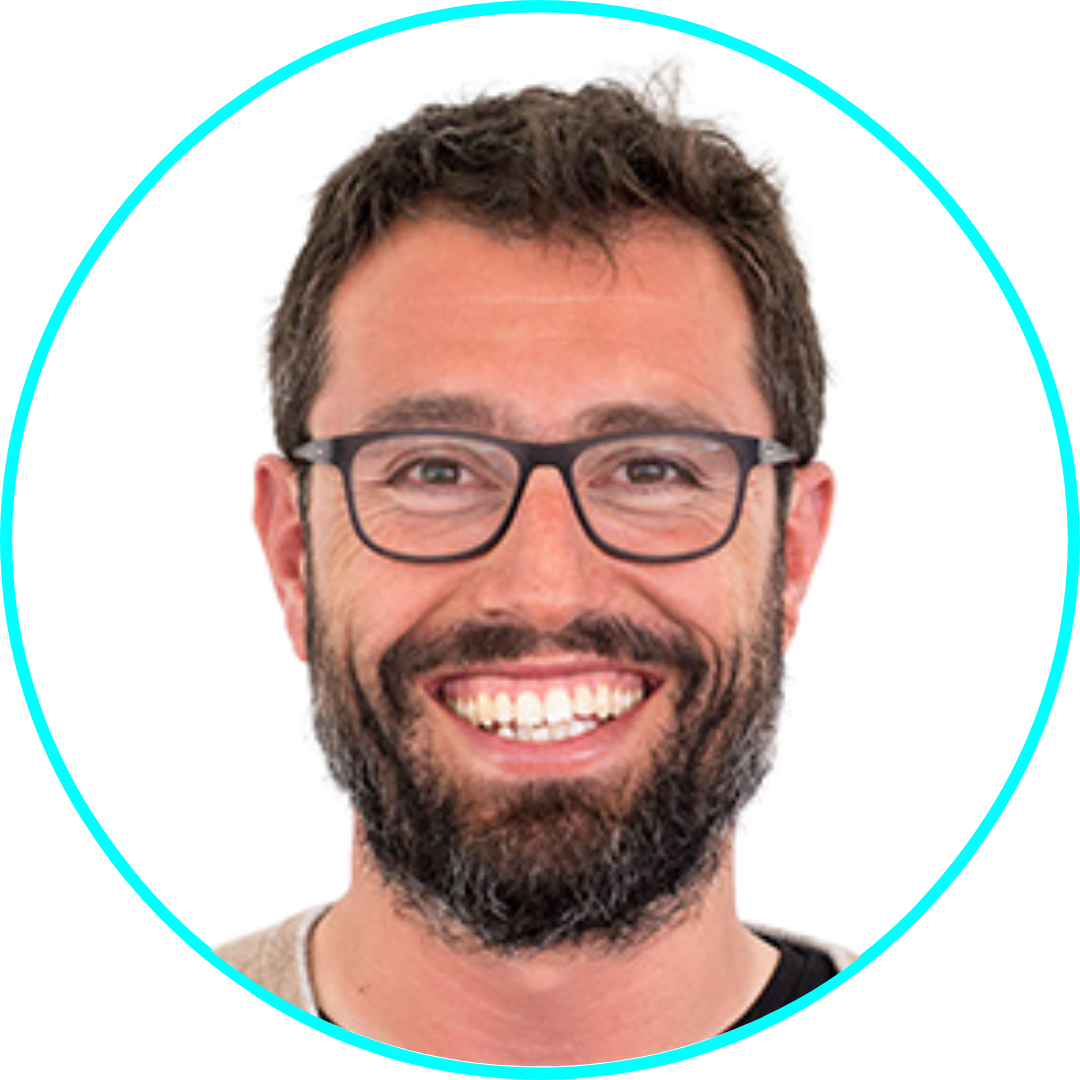
Dr. Luca BaldassarreLead Data Scientist,
Swiss Re
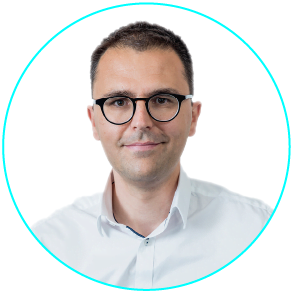
Dr. Marcin PietrzykCEO,
Unit8
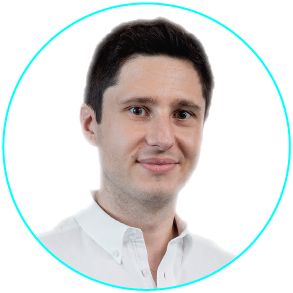
Michal RachtanCTO,
Unit8
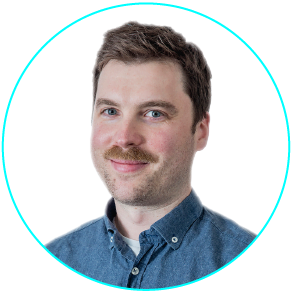