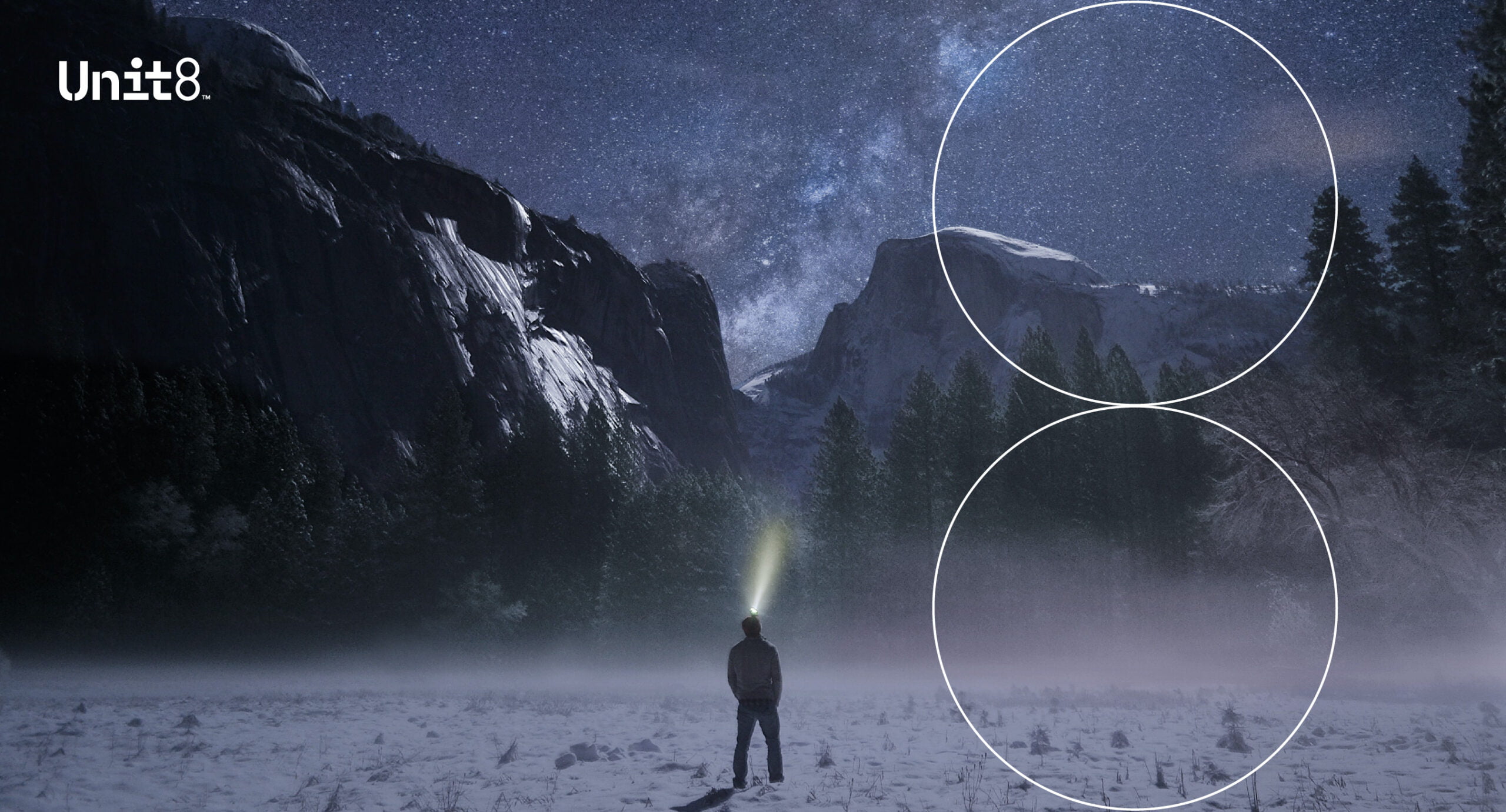
- Oct 20, 2021
- 8 minutes
-
Kamil Zalewski
-
Yohann Doillon
Overview
Selecting an AI project that delivers business value is a significant challenge for any company, but it’s especially difficult for companies choosing their first AI project.
Companies that lack AI experience often gravitate toward AI use cases that don’t align with their immediate business needs or use cases that bring unnecessary technical and feasibility risks. When these projects fail, the companies may find it difficult to continue investing in AI or to attempt subsequent AI projects.
I’m Kamil Zalewski, head of Unit8’s AI practice, and in this article, I’ll explain our best practices for selecting, sequencing, and executing a successful AI project at your company. By choosing the right use case, adopting an iterative approach, and choosing the right deployment model (buy, build, or partner), you can maximize your company’s chances of AI success—both now and in the future.
How to think about the problem
At Unit8, we advise companies to think about AI use case selection in three phases, each of which builds on the previous one. The first is understanding AI, the second is applying a systematic selection process, and the third is leveraging best practices to avoid common mistakes. I’ve organized this article around those same three principles:
-
Understanding AI in a business context.
Choosing the right AI use case for your company starts with understanding what AI is and isn’t good for, as well as what your company’s business needs are. We’ll start by explaining where AI can be most useful.
-
Methodology for selecting a successful AI use case.
The process of choosing an AI use case may be different for every company, but there are some common principles—such as dreaming big and starting small—that all companies should follow. We’ll outline those principles in this section.
-
Key takeaways and recommendations.
Many projects die in the planning stage. In our last section, we’ll provide a few takeaways and recommendations for getting your AI project off the ground.
AI business context
AI will have a profound impact across every sector. McKinsey and PwC estimate that AI will account for $13-15 trillion in global GDP growth by 2030, with $2.5 trillion of that growth coming from Europe.
The truth is that we already see and experience AI in our day-to-day lives. Whenever we use a voice assistant for example or browse movie recommendations from Netflix, we’re reaping the benefits of advanced AI research and technology.
At the same time, many traditional industries have not yet adopted AI. Think about agriculture, manufacturers, or chemical producers to name a few. These industries employ many millions, but they often lack the expertise and talent to adopt AI.
It isn’t just that these companies are reluctant to change. In many cases, they’ve already attempted to implement AI technology. But these projects have proven to be difficult to implement successfully, and the hype around AI has often made it more difficult for companies to choose the right projects.
From a technical perspective, AI is also poised to make rapid changes. Companies are collecting more data than ever before (both because storage prices have decreased and because their systems generate more data), and computing power is less expensive than ever before.
Four simple concepts to keep in mind before getting started.
-
-
Dream big, but start small.
AI can create significant business value for your company, in the long run, but don’t try to tackle everything at once. Gain experience by starting with a small proof of concept (PoC), and using the results and impact from that proof of concept to get organizational buy-in for future projects.
-
Focus on measuring value.
At the end of the day, business leaders want to be able to measure return on investment (ROI) from their AI projects. That means successful AI projects need to be integrated into everyday business processes and monitored, rather than being discontinued after the PoC is over.
-
Make sure you’re using AI to solve an AI problem, and that you have the right data available.
AI tends to require that you have the right type of data to use as inputs for AI models and that this data is clean and properly structured. In contrast to other types of innovative company initiatives, data availability is a core requirement for AI projects.
-
Choose a project that is both feasible and valuable.
Andrew Ng, an AI thought leader, points out that successful AI projects need to deliver business value and be technically feasible. Projects that don’t deliver value won’t be seen as successes, and projects that aren’t feasible will lead to project delays and cost overruns.
-

Characteristics of a successful AI project
Unit8’s AI project selection methodology
We’ve developed our AI project selection methodology over the course of more than 40 successful AI and machine learning projects for customers. There are other methodologies, but the following steps have proven most effective for us.
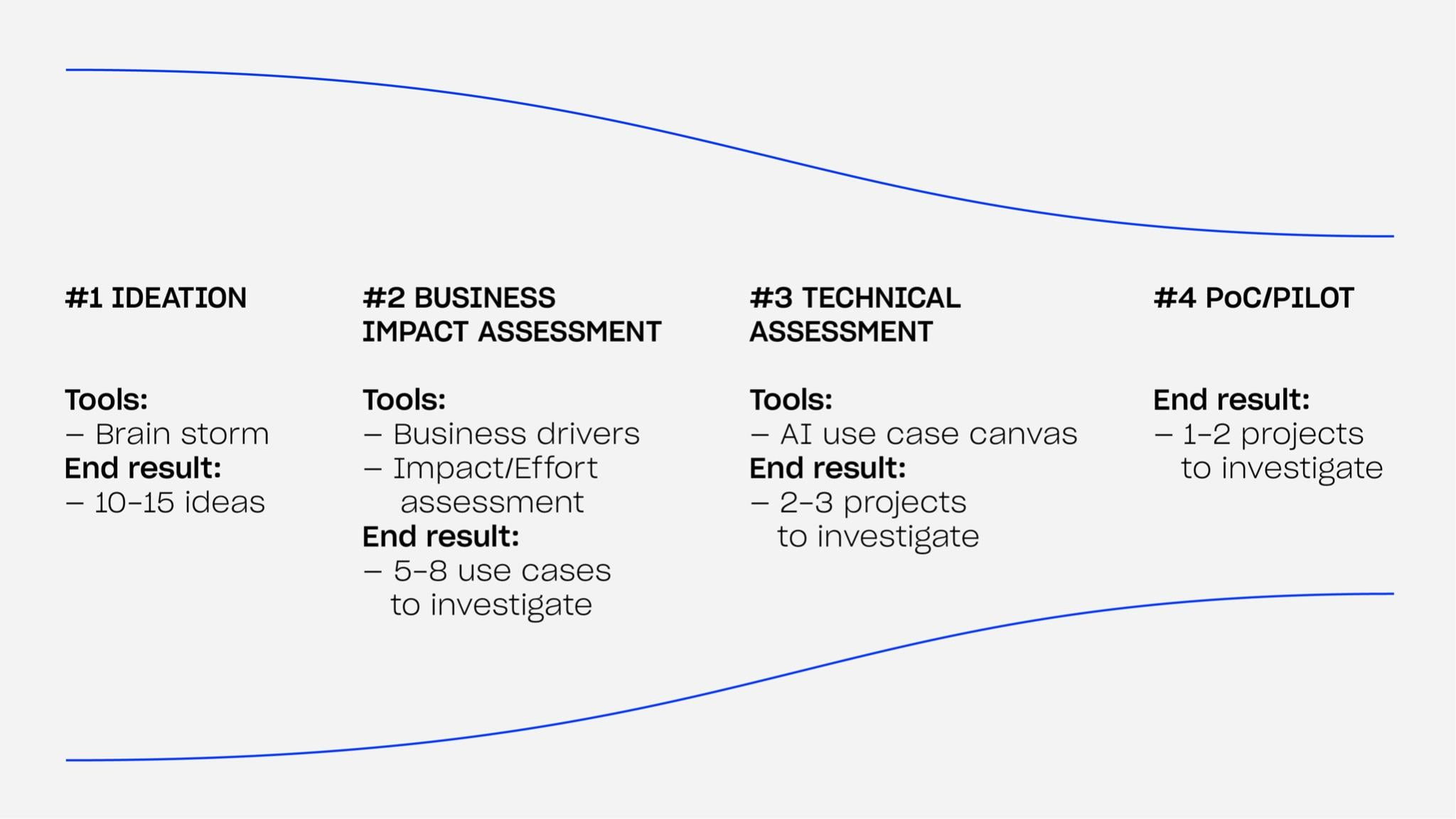
AI project pathway
Step 1: Generate a number of ideas.
The first step in choosing an AI project is ideation. You know your business’ key metrics and available data best. In this phase, you should brainstorm 10-15 ideas for AI use cases. There are no wrong answers in this phase. Your goal is to generate a variety of ideas for consideration. The key to a successful ideation phase is the focus. Ask stakeholders what pain points they feel most acutely, as well as how they measure success (e.g., increased revenue or reduced costs). Gain an in-depth understanding of what tasks can be automated, and what tasks require human input. A good problem statement or idea should identify both the underlying data issue and the metric for success. A few examples from recent projects we’ve conducted:
- A global chemicals producer struggles with hard-to-detect issues in manufacturing that slowdown production.
- An international insurance company is negatively impacted by inaccurate sales forecasts.
- A pharma producer is running out of production capacity and wants to reduce the CapEx investment needed for production to keep pace with demand.
- A Geneva-based manufacturer sees an increasing number of defects in the goods it produces and wants to reverse the trend.
By starting with a problem, identifying the impact you hope to create, and setting specific, achievable goals, you’ll ensure that all stakeholders are aligned on the scope and goals of the proposed ideas.
Step 2: Conduct a business impact assessment.
After you’ve generated a large list of ideas, it’s time to look at business drivers and assess the costs and benefits of various projects. In a manufacturing context, for example, it might make more sense to work on a project that requires access to only a small segment of factory operations, rather than undertaking a project that requires significant behavior change across an assembly line. At the end of this phase, you should be able to narrow your 10-15 ideas to 5-8 discrete use cases.
A few ways to verify that your AI use cases will deliver a business impact are to see if they align with the following business drivers:
- Reduced costs
- Increased revenue
- Risk reduction
- Launching a new data product (i.e., creating a new AI-driven product)
After you’ve linked use cases to business drivers, it may be helpful to use the following impact-effort matrix to properly scope the project.

AI project impact/effort assessment
Step 3: Assess technical feasibility.
Many businesses overestimate what can be accomplished with an AI POC. Make sure you verify technical feasibility for your 5-8 proposed use cases by asking technical experts whether you have the right data for the project, the right in-house AI/ML personnel to conduct it, and what a reasonable timeline for the project might be.
At Unit8, we use the following AI business canvas to make sure that we’re on the same page as our clients when it comes to what a project might cost, how long it will take, and how success will ultimately be measured. We find that building the AI business canvas sparks a lot of discussions early on and simplifies the process of building a business case or a larger proposal down the line.

AI business canvas
Step 4: Run a proof of concept or pilot.
When it comes to running a successful project pilot, our recommendation is to use an Agile-based methodology (which is iterative, rather than relying upon a waterfall-type set of requirements), and aim to create a successful minimal viable product (MVP) that can be moved into production at the end of the POC.
One question we often hear is how to decide whether to buy, build, or partner in order to deliver a successful AI implementation. There’s no one-size-fits-all answer, but here are the questions we advise companies to ask when making this decision.
Questions to ask when considering buying an AI solution:
- Can my company buy an “off-the-shelf” solution?
- Does the “off-the-shelf” solution match my company’s needs, or is significant customization required?
Questions to ask when considering building or partnering on AI implementation:
- Does my company have the requisite AI expertise and talent in-house?
- Does my company have the in-house AI experience and capacity to deliver on this project?
- How quickly do I need the AI solution?
- Does the project require significant domain expertise?
Key Takeaways from Successful AI Projects
Choosing an AI use case is important, but execution during a POC matters just as much. Here are keys to successful AI execution that our team has observed over the course of dozens of successful AI/ML projects
- Focus on quick wins. Look for projects that can be delivered quickly and have high chances of success.
- Have a plan for proving value at each step of the pilot. Use a proof of concept to get buy in and pave the way for a larger AI project.
- Focus on business problems. Projects associated with pressing business problems have a higher chance of success early on and expansion, in the future.
- Bring in SMEs early on. Make sure your AI experts work hand-in-hand with domain experts so that they stay on the right track.
- Be pragmatic. A good machine learning model that runs in production is better than a perfect model that can’t be deployed.
- Communication and change management are key. Make sure the project gets the right visibility (from beginning to end) from business leaders to ensure continued momentum.
What’s next
Selecting an AI project is an exciting exercise, but it usually is one of the first steps of a much longer journey. In the Data & AI journey article, we guide you through the typical AI adoption path that will take you from gaining awareness all the way to leveraging the full capabilities of data & AI at an organization level. There, we are outlining our best practices and insights so that you can improve your AI capabilities regardless of your maturity stage.
Interested in working with us to help you select a successful AI project? Reach to discover how we conduct ‘AI discovery workshops’ to facilitate the process.
You can trust us
Unit8 is a Swiss data and AI solution provider that helps companies bridge the gap between their data and the value that this data can unlock. We focus predominantly on non-digital native industries, or industries that have been traditionally underserved by advanced data and technology solutions. We bring expertise and best practices from some of technology’s most innovative companies, including Google, Amazon, Microsoft, Palantir, and Apple.
Error: Contact form not found.