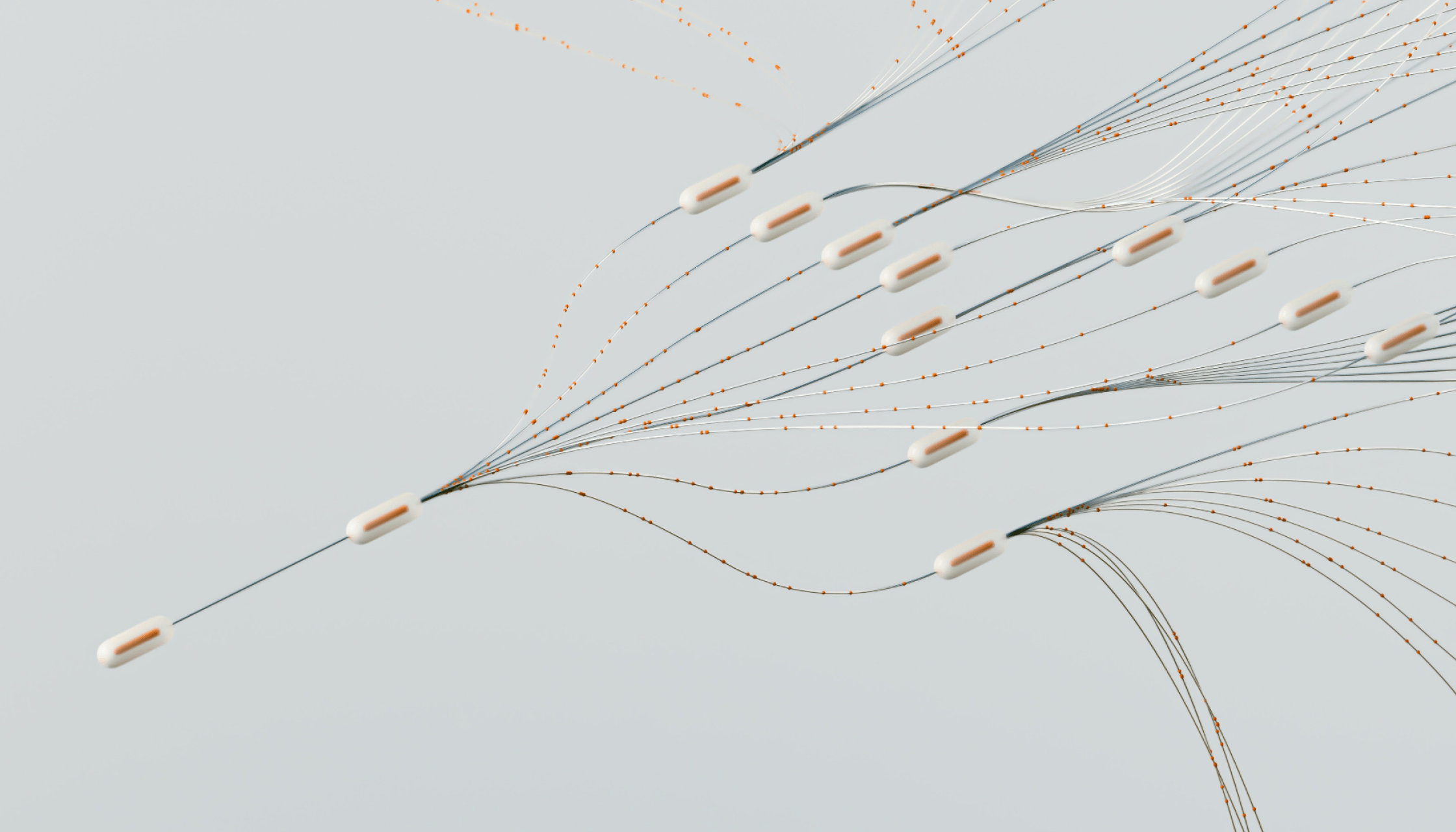
- May 10, 2023
- 10 minutes
-
Thibaut Paschal
-
Andreas Blum
-
Yohann Doillon
In today’s world, data is the most pervasive and valuable asset.
Ensuring robust data governance around integrity, confidentiality and availability, as well as ownership enables companies to both…
- be compliant with regulations and manage operational & reputational risks;
- be competitive by unlocking data analytics at scale to increase efficiency, make data-informed decisions and generate new revenues.
This article addresses the “be compliant” aspect with the help of a framework and launches a new series that will uncover and simplify data governance.
Introduction
What is Data Governance, and why does it matter?
“Data Governance is a system of decision rights and accountabilities for information-related processes, executed according to agreed-upon models which describe who can take what actions with what information, and when, under what circumstances, using what methods.” – The Data Governance Institute
Data governance as a business practice is gaining traction due to two main driving levers. First, as the list of data privacy regulations continues to expand (e.g., GDPR, FINMA, GxP, etc.) organizations are under more scrutiny than ever before to have a solid grasp of the data they are using. Second, as organizations rely more and more on data analytics to drive crucial business decisions, that value-add is under risk if the data consumed is compromised. Companies can minimize their risk on both fronts by properly managing the availability, usability, integrity and security of their data. Effective data governance ensures the data is trustworthy, consistent and will not be misused.
The North Star
What does “good” data governance look like?
Data governance is good when its end-result fully ensures the company against applicable data privacy regulation at various levels of granularity (group-wide, divisions, functions, data domains, etc.). The three main pillars of a robust and effective data governance program are depicted in the diagram below.
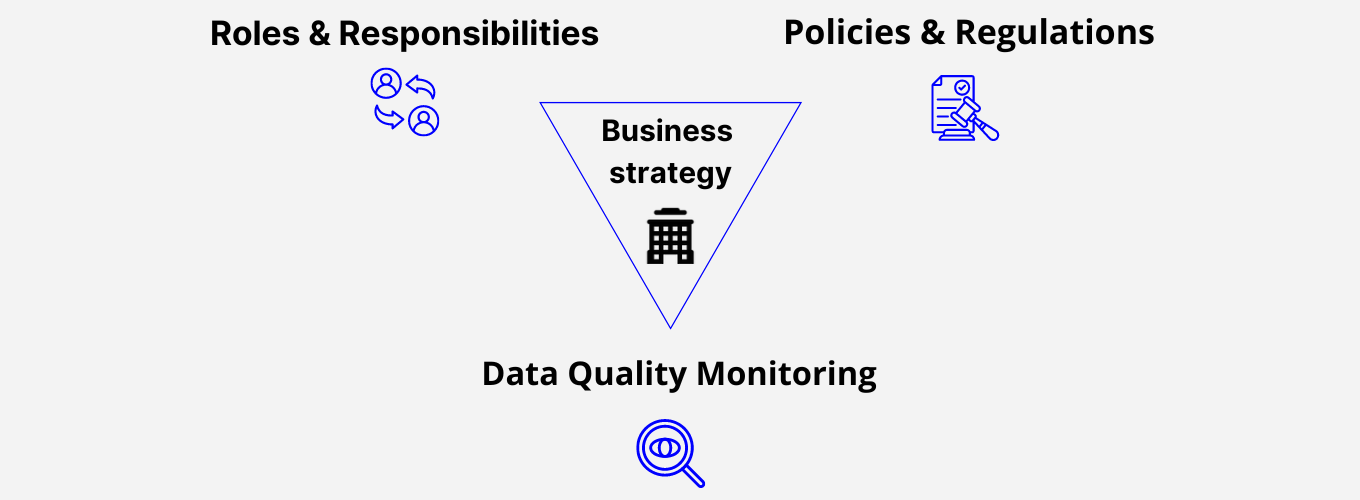
Figure 1: The three pillars of data governance
Roles and responsibilities
First, it is key to ensure accountability by setting up governance forums, defining clear roles & responsibilities, and defining escalation paths across the organization (e.g. Group CEO, Divisional CDO, Data Owner, Data Steward).
The caveat here is that although the standards and policies are defined centrally, local domain teams still have full autonomy, and accountability to execute these standards in the most appropriate manner for their particular domain. For this reason, we recommend following a federated organizational model (hybrid between centralized and decentralized models).
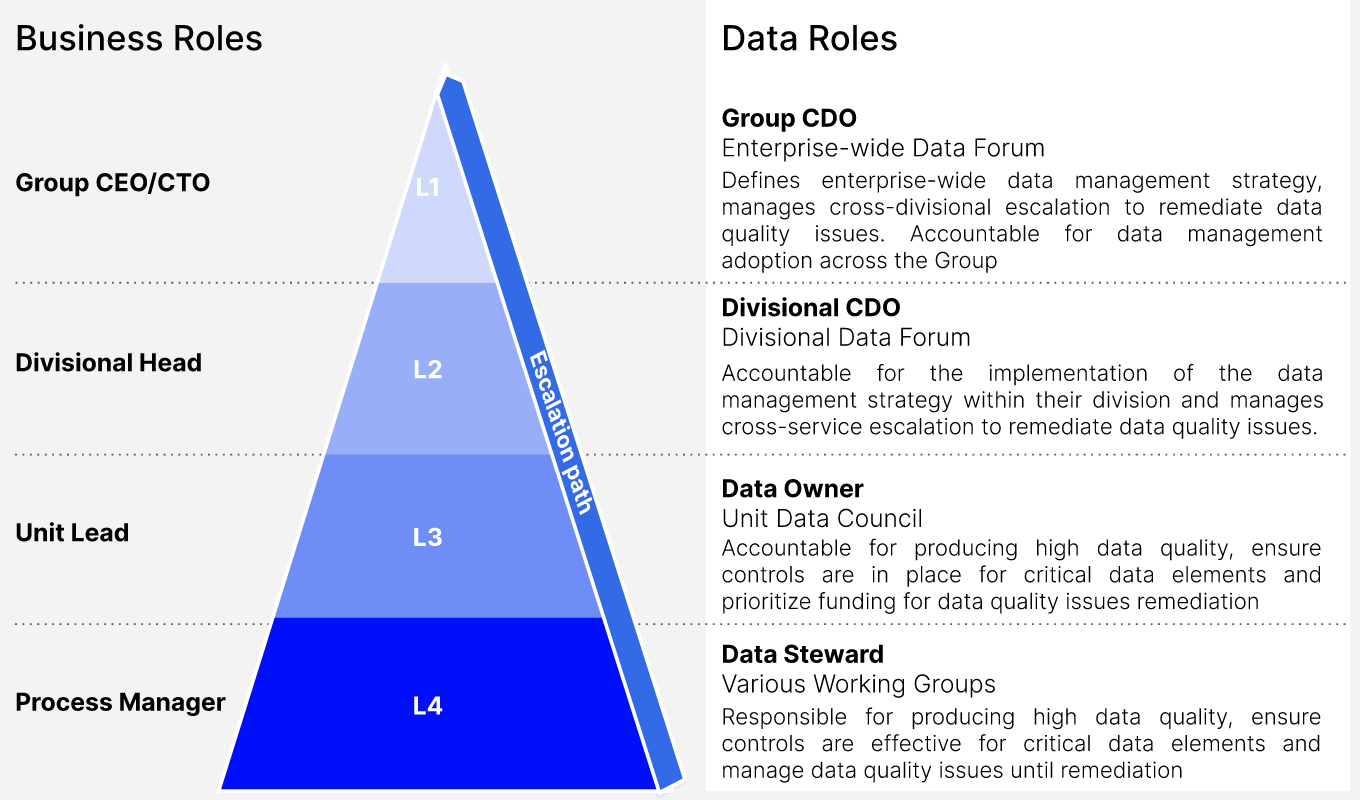
Figure 2: Roles and Responsibilities
Policies & regulations
Second, defining, communicating and enforcing company policies creates a culture of transparency, consistency and compliance regarding the utilization of data. Defining exhaustive and effective policies requires the use of plain language, gathering employee feedback and regular updates. Critical for AI & data topics, especially considering the speed at which new terminology and concepts appear are policies that are understandable and correct (i.e., reflecting the current state of AI).
To enforce policies, we encourage teams to regularly communicate in intervals, leverage multiple channels and encourage employee feedback. Without effective policies and its enforcement, companies run the risk of data leakages (internal and external) and subsequently damaging their competitiveness and/or public image.
Data quality monitoring
Third, we also recommend proactively controlling the quality of your critical data assets using data quality monitoring capabilities. Once critical data elements are identified, data quality rules must be defined and implemented via dedicated tools along major data dimensions (correctness, completeness and timeliness). Data consumers can be proactively alerted when data quality and associated risk threshold are breached enabling organizations to manage operational and reputational risk, as well as to show evidence of good data management to regulators. Such monitoring capabilities (see Figure 3) allow CDOs (Chief Data Officers) and other data decision-makers (e.g. data owners, domain owners) to consistently monitor data quality, quantify their risk versus business impact, and take preventive actions. The dashboard below shows a blueprint of how Unit8 tracks different metrics across domains, along with relevant alerts and filters.
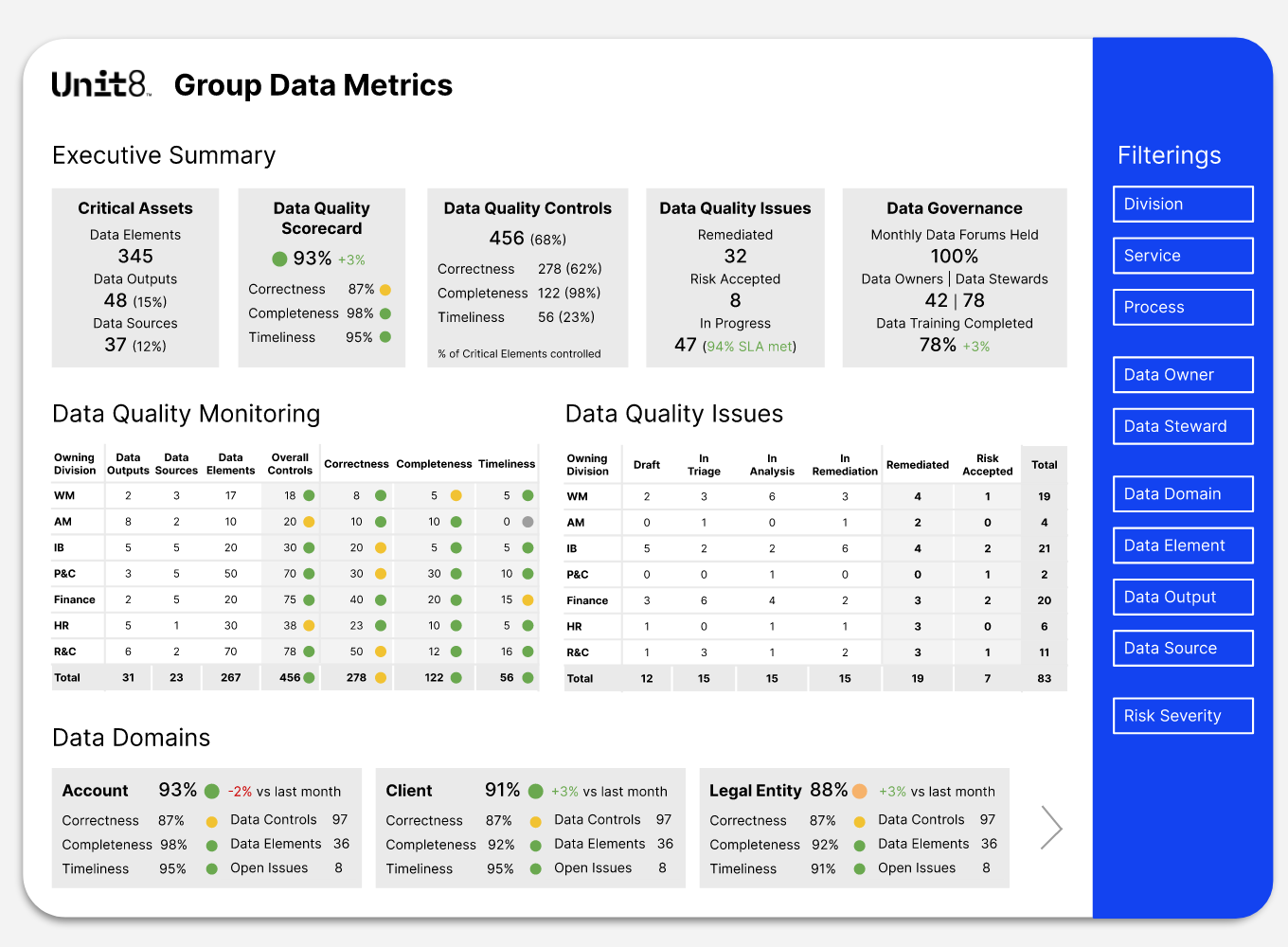
Figure 3: Unit8 blueprint Data Quality Monitoring dashboard
Unit8 Data Governance Framework
What are the best-practices for practical implementation?
Our Data Governance Framework is designed on the one hand to ensure regulatory compliance whilst on the other hand enabling organizations to derive maximum value out of AI and analytics, whilst keeping risks in check. We see the following elements playing a key role in the framework:
- WHAT – Once a policy has been agreed upon, Chief Data Officers must appoint data role holders to ensure accountability.
- WHO – Governance bodies must then be set up to cascade down the strategy, steer the execution of data governance activities and resolve escalations.
- HOW – Becoming compliant requires the execution of various inter-linked activities, up to the identification of critical data elements, implementation of data quality rules for relevant dimensions, down to data consumers of business risk due to low data quality using monitoring dashboards.
- TOOLING – Leveraging the right tools can support organizations in developing a data culture, educating employees in producing data of high quality, and overall decreasing operational and regulatory risks of not being compliant.
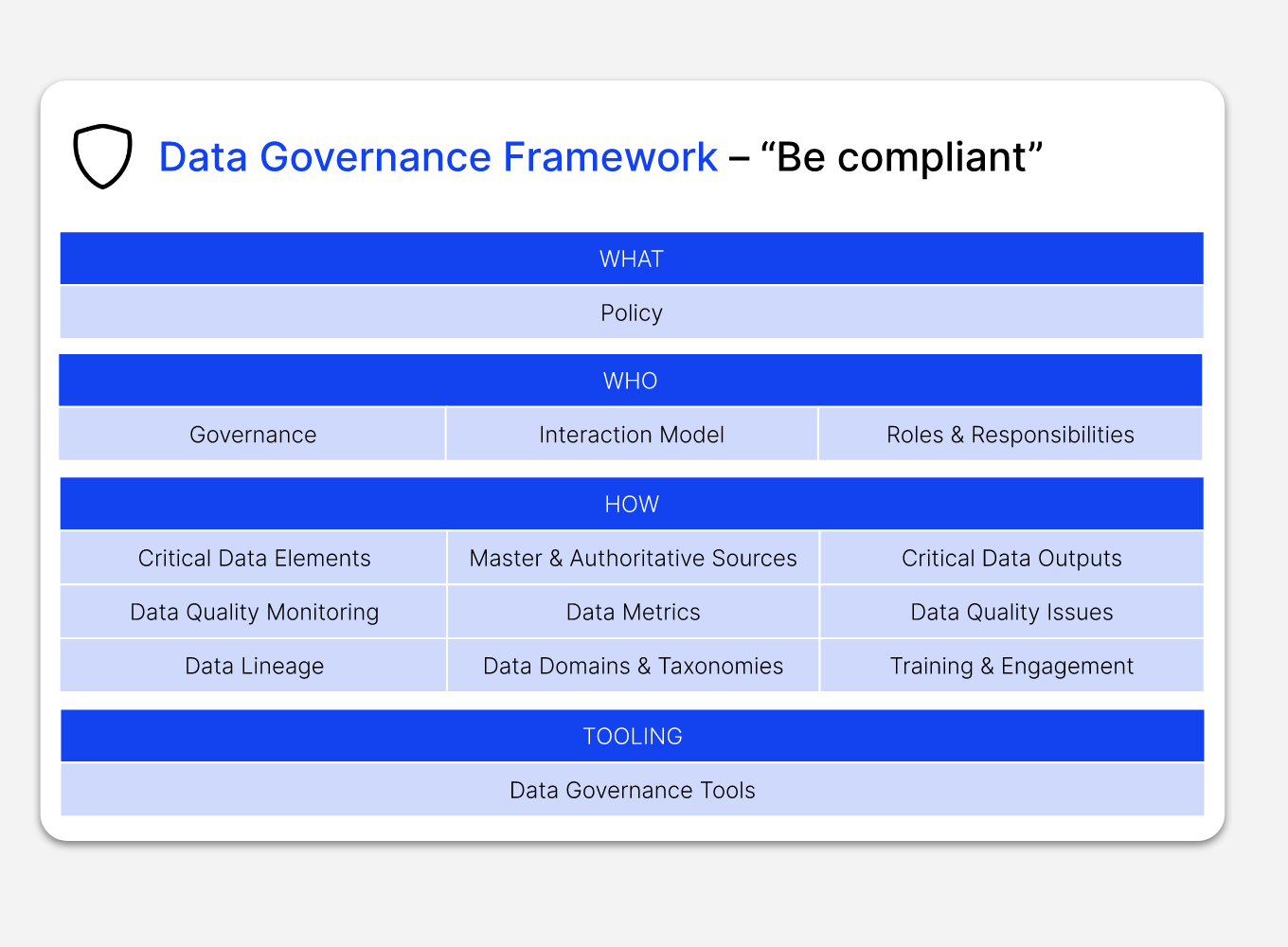
Figure 4: Unit8 Data Governance Framework. Part 1 - ‘Be compliant’
Establishing Data Governance bodies
In order for organizations to follow a federated data governance approach, standards and best practices should be defined centrally by the Group Data Management Office, but accountability should sit within Divisions where activities will be executed in the most appropriate manner for their specific domain and regulations.
Various data governance bodies and execution teams steer the enterprise-wide data management implementation with varying levels of responsibilities.
- The Group Data Forum is chaired by the Group Chief Data Officer and responsible for defining the group data strategy, steering the execution of data management activities, managing budget & resource, as well as resolving cross-divisional escalation to remediate data quality issues.
- Regulators-aligned Data Forums are cross-divisional forums which are responsible for the compliance of the organization towards local regulations (e.g., FED, FINMA). For large and global corporations, having a dedicated regulator-aligned forum can tremendously help to steer divisions which are under this jurisdiction in becoming compliant.
- Divisional Data Forums are accountable for the implementation of the data management strategy within their division and manage cross-service escalation to remediate data quality issues. Divisions must have dedicated resources to support data owners and stewards in implementing data management capabilities and ensuring best-practices are used across a single division.
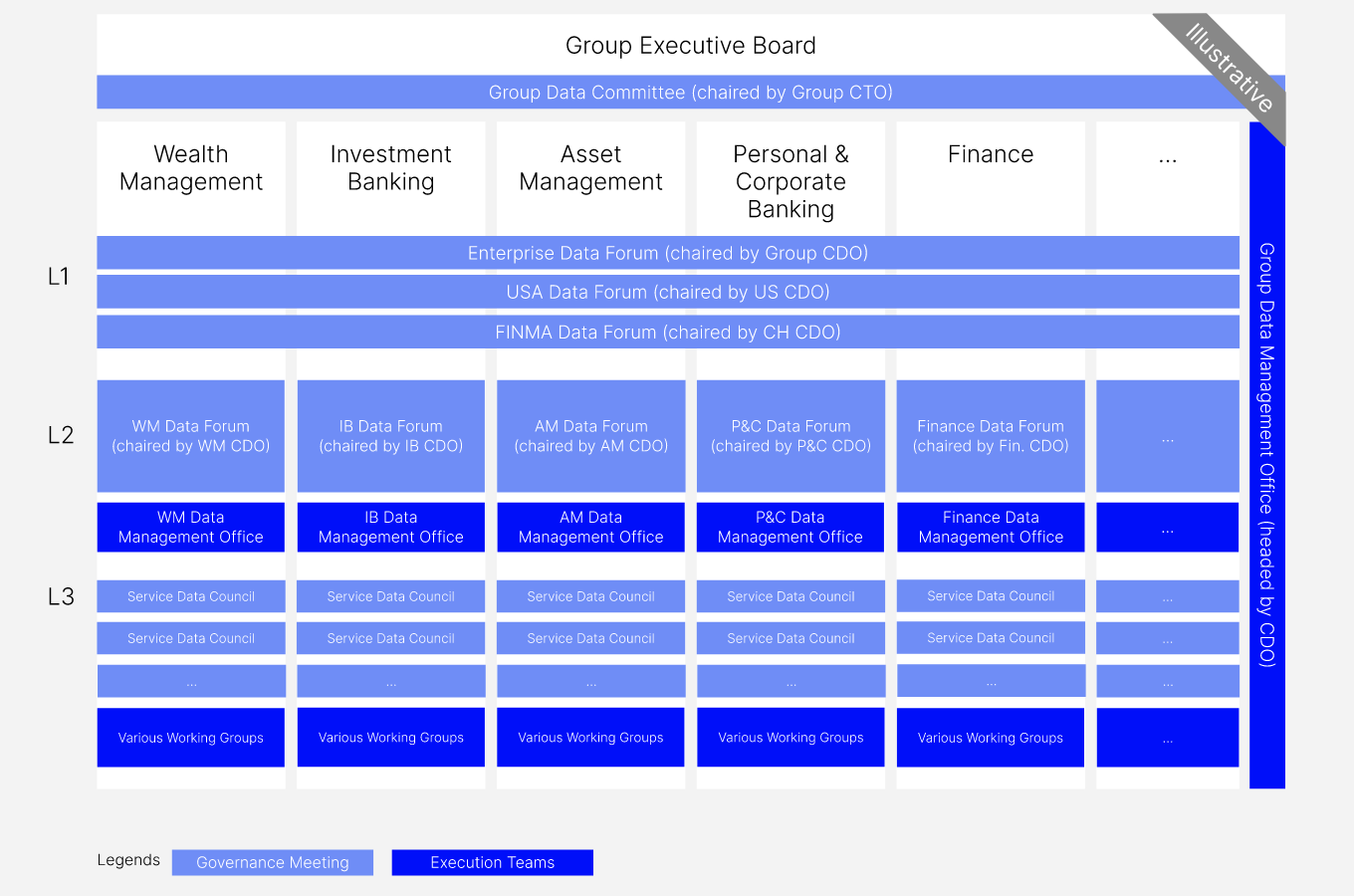
Figure 5: Data Governance bodies across an organization
Implementing data quality monitoring and mitigating risk via alerts to data consumers of ‘low’ data quality
Monitoring data quality enables organizations to proactively control the quality of their critical data elements. From our experience, companies commonly adopt a reactive resolution approach to data quality issues, which leads to increased risk on both financial and reputational aspects. Therefore, we recommend becoming proactive by building data quality rules along major data dimensions (correctness, completeness and timeliness) of critical data elements. Organizations can either choose to build in-house data quality rules on their data pipeline or leverage all-in-one vendor tools (e.g. Talend, Informatica, etc.).
As described below in Figure 6, a successful implementation should encompass both a technical and business integration. Data consumers should be proactively alerted, business impact measured as part of the rule implementation, and finally data publication could potentially be interrupted if the risk breaches a defined threshold. With such a solution, not only can organizations decrease operational, financial and reputational risk, but also evidence good data management adoption to regulators.
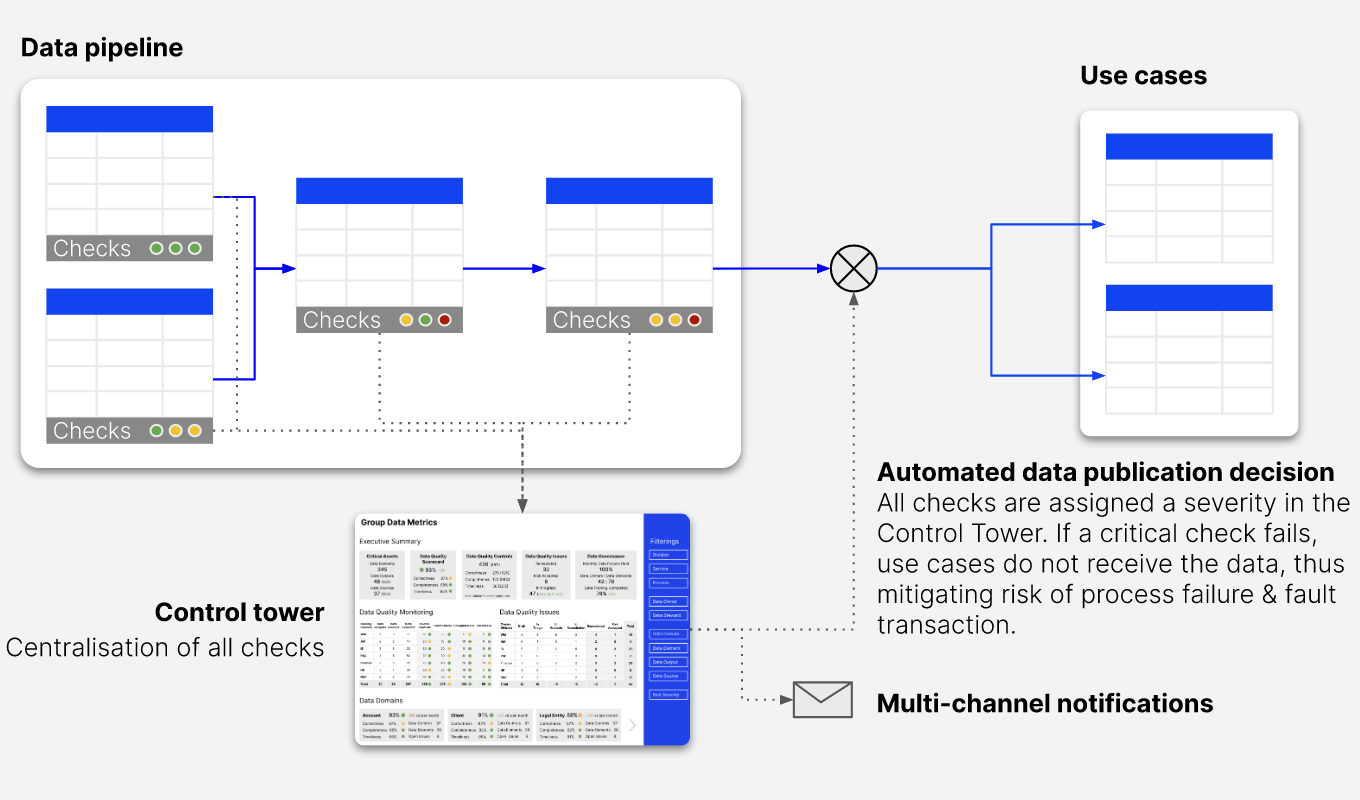
Figure 6: Sample end-to-end data quality monitoring project with rules, control tower, alerting & data refresh downstream
Implementation risks
What do I need to be aware of and mitigate?
In our experience, the following top 3 risks are likely to arise during implementation:
1. Siloed data governance efforts
More often than not the IT department is silently voted to be the de facto arbiter of governance programs. The common preconception found among non-IT employees is that data governance is a technical problem and should, evidently, be handled by IT. This is incorrect; data is owned by the business, not by IT. It is vital, from the very beginning, to rewrite the narrative and repeatedly communicate that data security & governance is a company-wide effort. Hence, every single employee must be held accountable for the misuse of data, and the responsibility does not solely rest on the vigilance of IT.
2. Misalignment of data governance and business plans
It’s easy to lose sight of the bigger picture when setting up a data governance program. Many mistake its goal to only be a harmonization of technology, policies and data management. Business strategies need to be at the center, making sure that the governance programs serve to achieve the goals. This can be achieved by including top executives in the governance formulation and asking them to communicate their conviction company-wide.
3. Neglecting trust-based security
Cybersecurity and data governance go hand-in-hand to strengthening a company’s data security. Companies often overlook their complementary interconnection, often failing to perceive both as two sides of the same coin. We hence encourage binding in cybersecurity early during the development of data governance programs while allowing for a two-way dialogue to flow, ensuring each side gets their concerns and requirements heard.
Conclusion
To become compliant with regulators and set strong foundations for unlocking value from AI & Data Analytics at scale, data must be governed, owned and of good quality.
To summarize, we advocate implementing the following three suggestions:
- Secure buy-in from senior leadership and set governance bodies to steer data management adoption
- Ensure clear definitions of different data roles and responsibilities at all levels of the organization
- Systematically identify critical data elements, with a strict control of all major dimensions with data quality rules, ensuring issue remediation at source
The Unit8 approach enables companies of all sizes to proactively monitor data quality & risk, ensuring regulatory compliance, and supporting their Data Science journey at all levels of maturity.
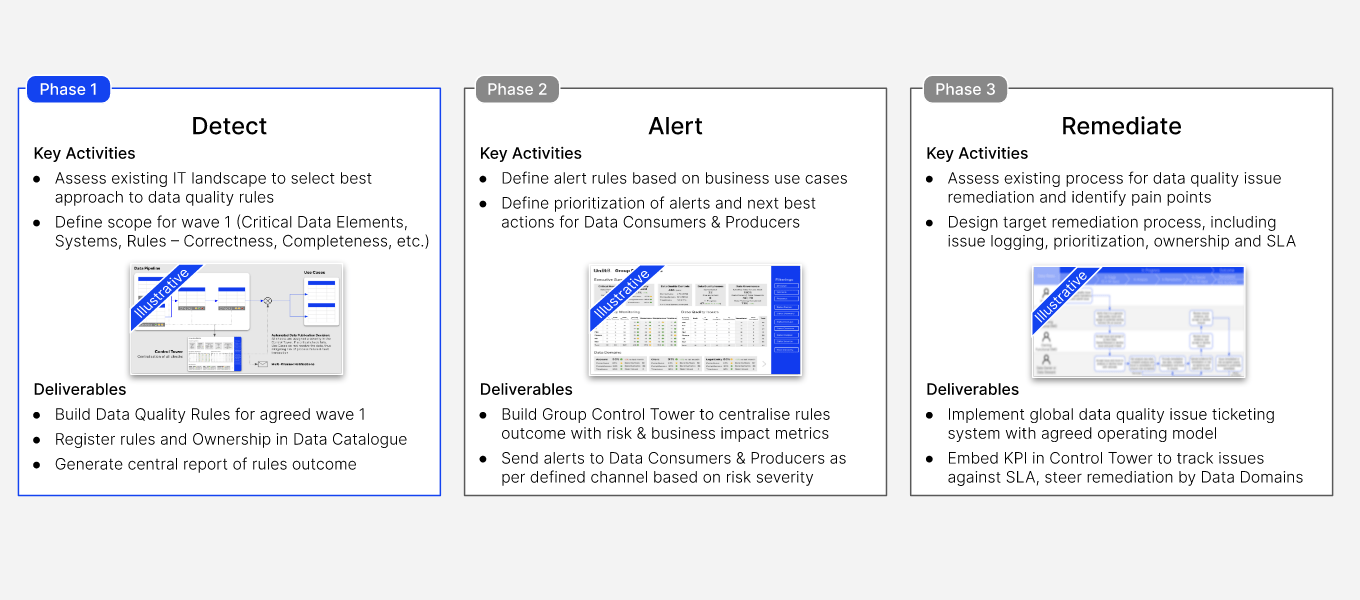