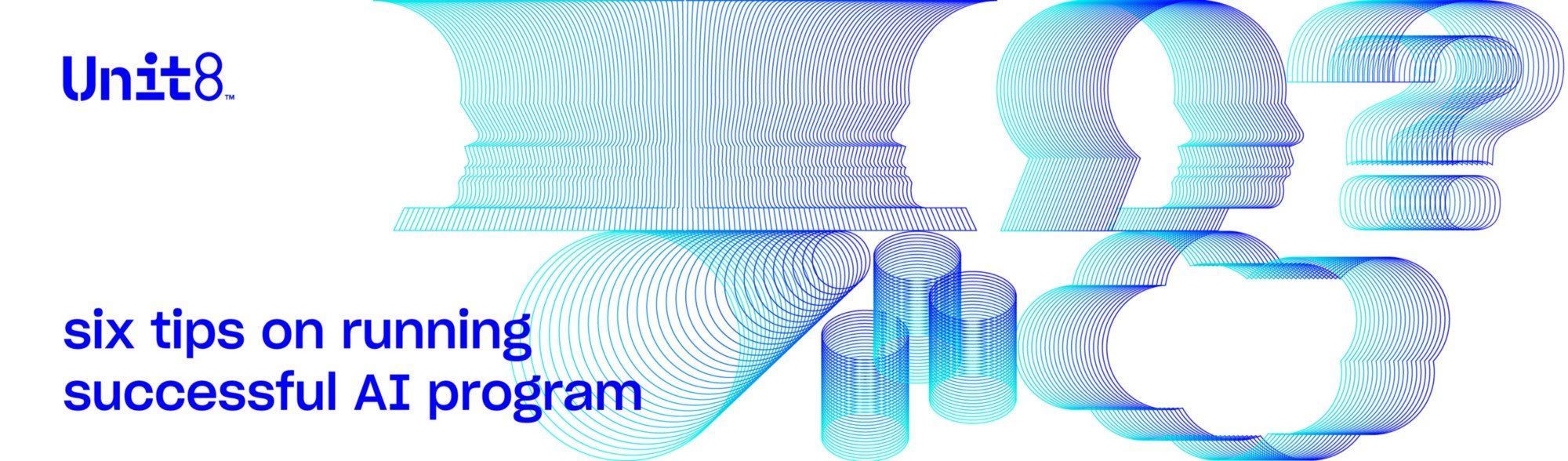
- Jul 6, 2021
- 7 min read
-
Kamil Zalewski
Introduction
Perhaps no technology has as wide a range of outcomes as artificial intelligence (AI). Properly deployed, AI solutions can help companies improve efficiency in manufacturing, identify company risks, and maximize revenue, among other outcomes. At the same time, a poorly scoped or implemented AI project can cost an enterprise millions and still not deliver a positive return on investment (ROI).
The best way to set your company up for AI success is to make sure you’re familiar with industry best practices from Day 1. In other words, it’s to make sure that you have the right people, processes, and technology plan in place — even before you get started. But what does that look like in practice?
Over the last couple months, I’ve been posting six hard-won AI lessons that the Unit8 team has learned over the course of dozens of successful AI deployments at scale. This experience has been gained as well in my previous role of head of analytics & AI in large Swiss telecom where Ive overlooked number of advanced analytics initiatives.
These principles are:
- Put business impact first
- Treat data as an asset
- Manage (inflated) expectations
- Get talent right
- Use a strong infrastructure foundations
- Don’t forget the business translators
#1: Put business impact first
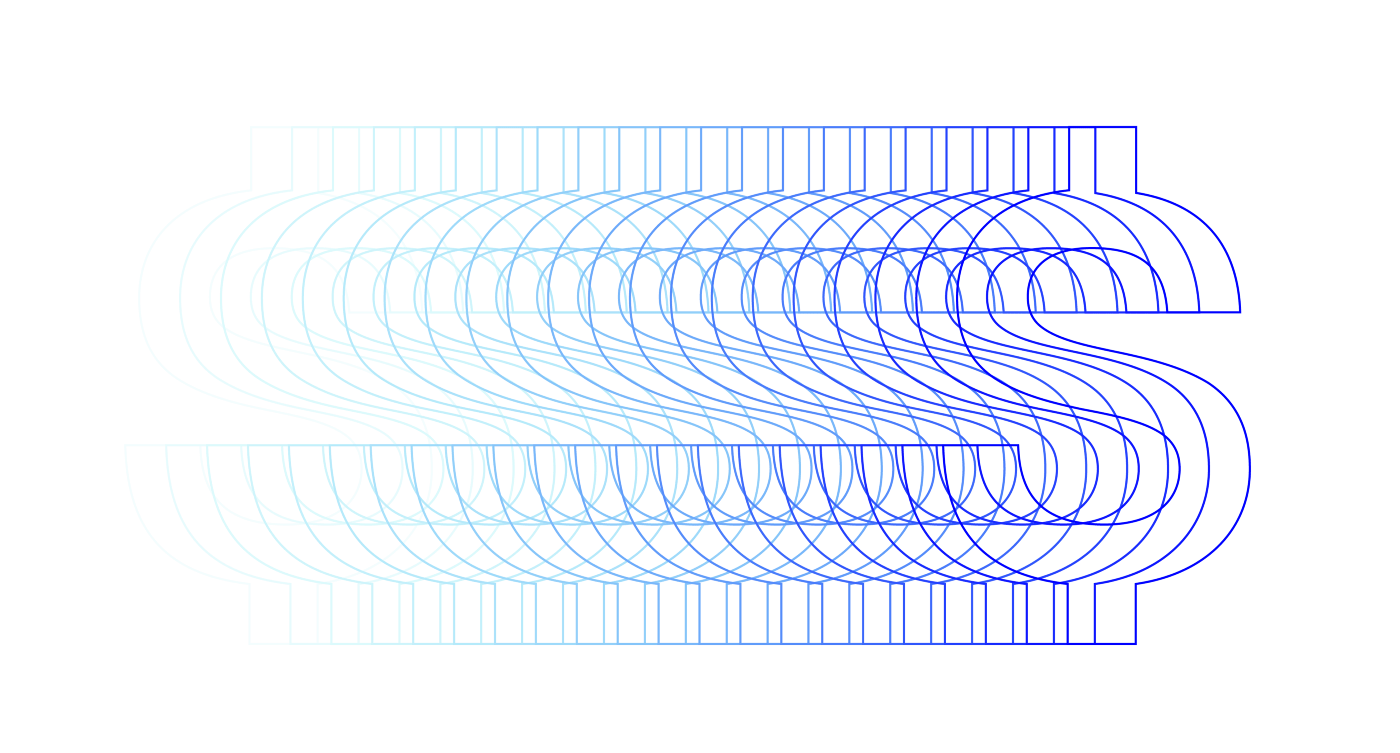
The first and most important AI principle is to start with a core business problem that you want your AI or analytics solution to solve.
As CEO of Unit8, I can’t tell you how many times I’ve heard corporate leaders try to start with a technology, rather than a business problem, in making AI investments.
If you’ve tried that approach, you know that choosing a technology first — rather than a business problem — is likely to go poorly. When it comes to analytics and AI, there’s rarely a one-size-fits-all approach.
That’s why we recommend starting with a core business problem, evaluating different teams and technologies against their ability to solve that problem, and then testing your hypothesis with a pilot project.
By keeping a business outcome in mind from the outset, you can bring a level of accountability and focus that keeps your AI program on track at every step.
#2: Treat data as an asset
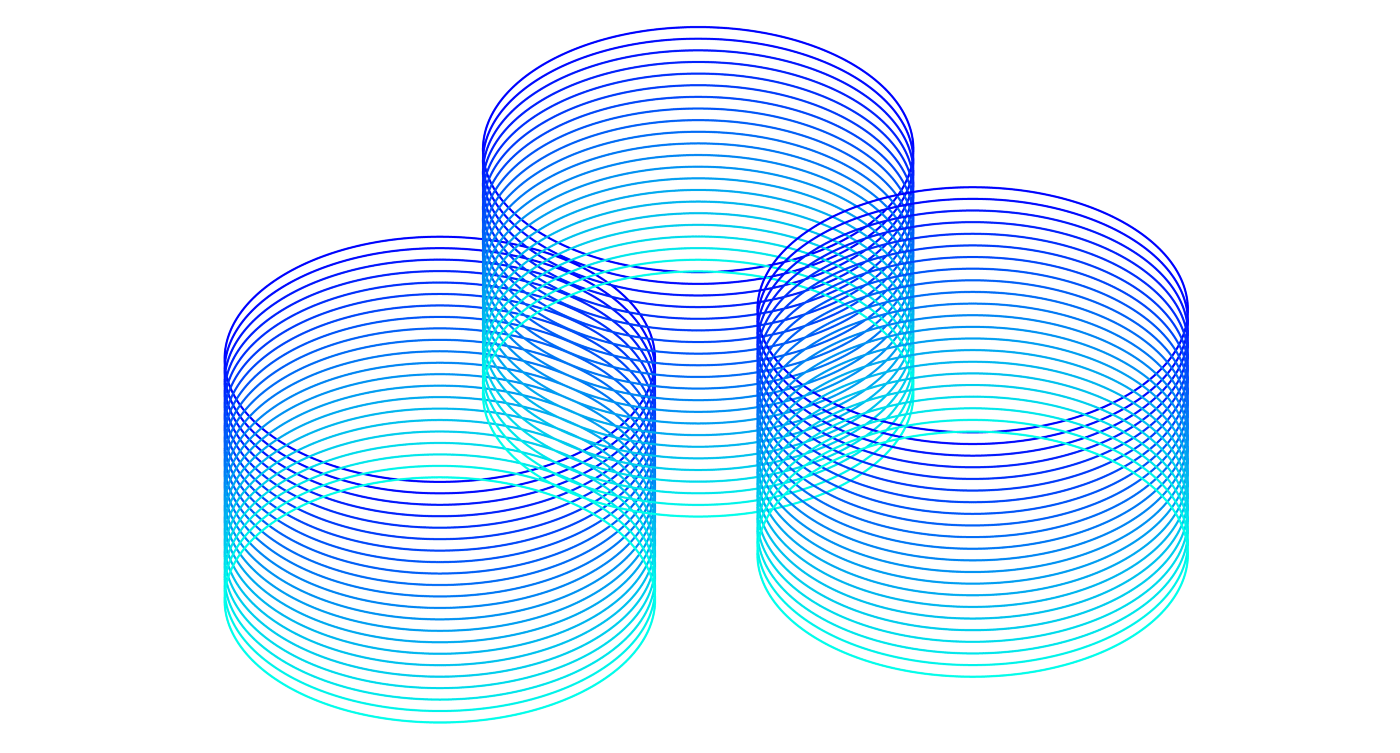
Today’s successful companies invest in accounting staff, finding top-tier CFOs, and implementing sophisticated ERP systems. Why? Because they know that they can maximize their profits by keeping a close eye on every company expense.
Unfortunately, few companies treat their data with the same care. Business leaders often say that “data is the new oil,” but they fail to treat their data like the precious resource that it is.
At Unit8, we’ve been surprised by how many large companies haven’t integrated datasets from across their company in a central location. That’s why we recommend that every company should think critically about how it collects, stores, governs, and manages data assets before it begins implementing AI solutions.
You can’t implement AI successfully if your data lives in silos. (Just listen to Novartis CEO Vas Narasimhan, who talks in this a16z podcast about the difficulties of bringing data together at a global pharmaceutical company.)
#3: Manage (inflated) expectations
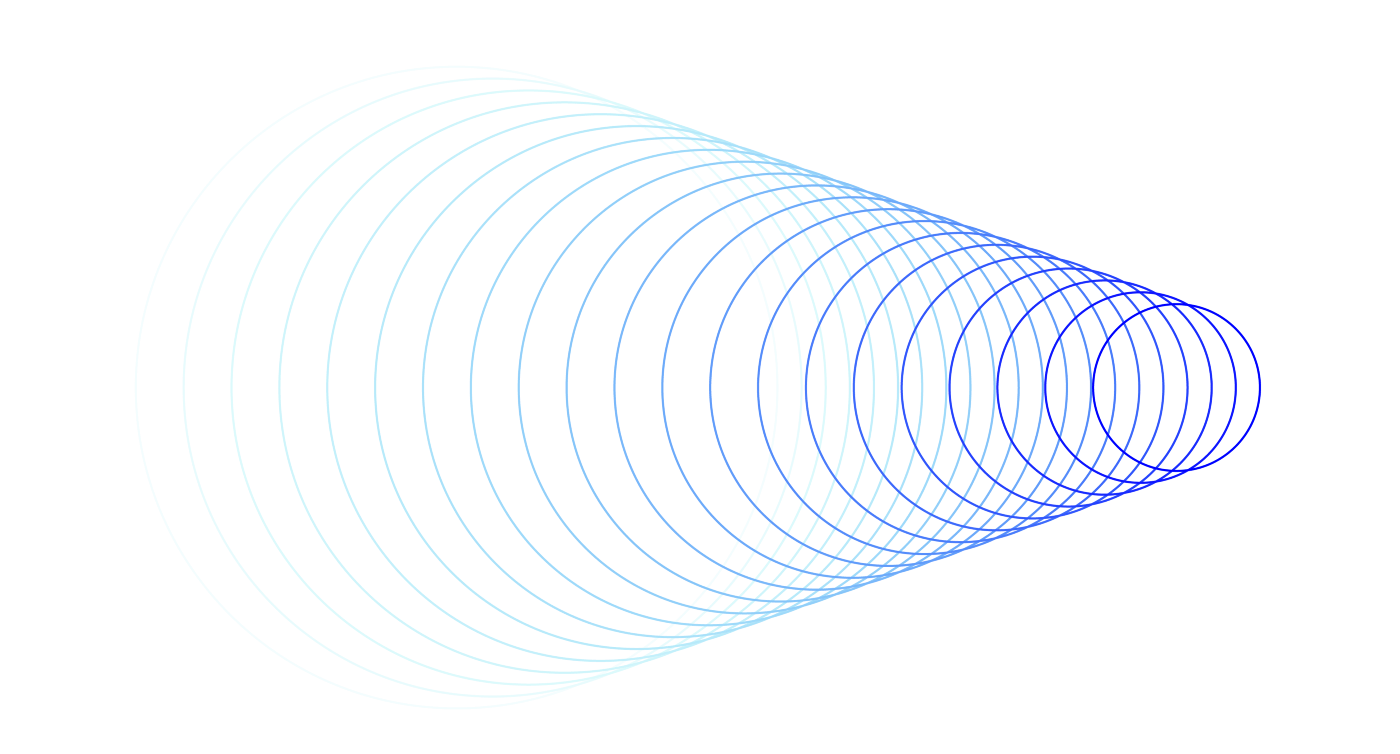
AI has been one of the most hyped topics in recent years. Unfortunately, the hype around AI has also fueled misconceptions and inflated expectations.
Consultants and publicists are often quick to talk about what AI can do, but often unable to deliver on the promise. That puts business leaders in a difficult position — to succeed with technology, they need to identify use cases for which AI is both effective and pragmatic.
Our advice for business leaders is simple: recognize that what seems too good to be true often is. Use common sense and start with feasible projects, rather than moon shots.
For AI and advanced analytics project managers, we recommend investing time and energy in customers education early on. That way, you can set the right expectations and avoid client disillusionment.
AI can lead to significant business outcomes, but only if clients and project teams base their decisions on reality, rather than hype.
#4: Get talent right
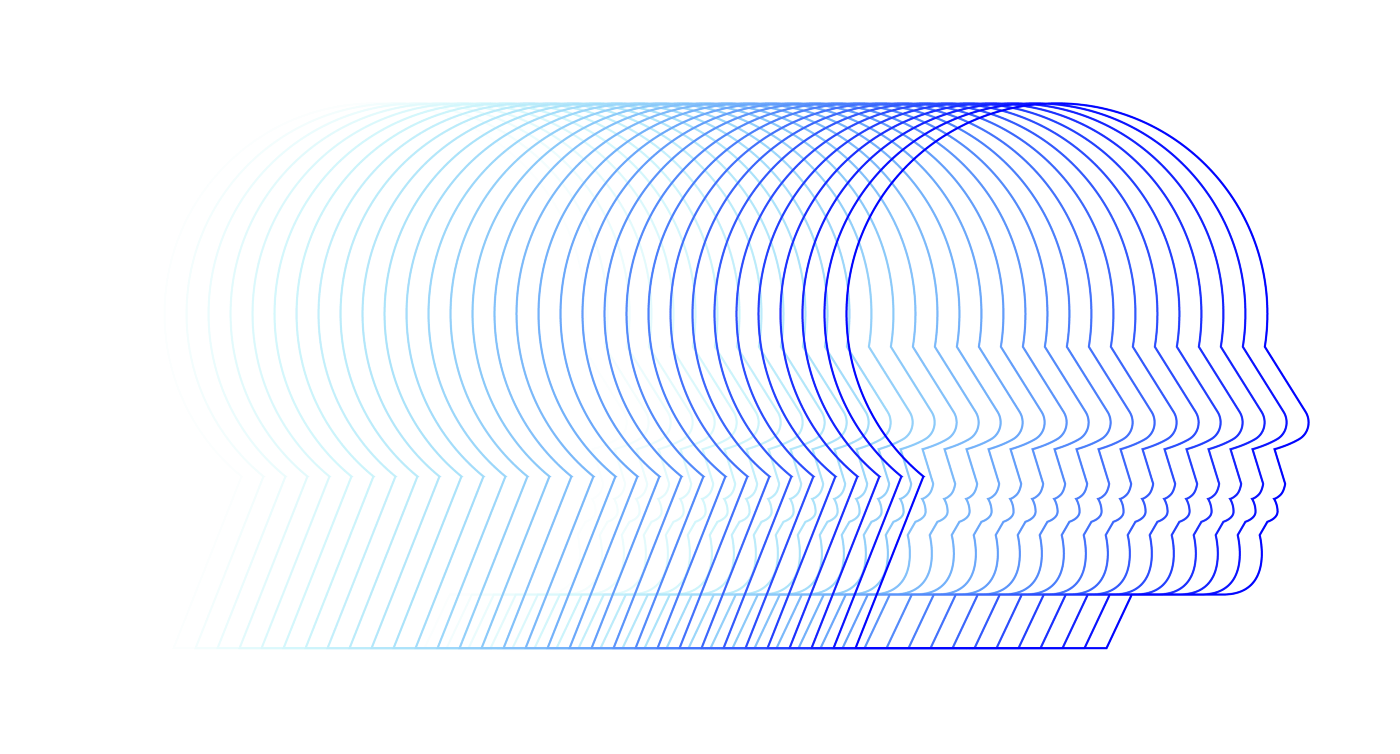
In most industries, the gap between average talent and top talent may not lead to significant difference in business outcomes. But when it comes to digital talent, choosing the right personnel is often the different between AI or analytics success and failure.
Many companies in the traditional industries underestimate the importance of finding the right talent. They may assume that it is enough to “hire a few data scientists” and point them at their business or data problem.
Here’s the new reality: in an era where powerful software solutions are free (e.g., open-source) or available via the cloud, it is often digital talent — not technology — that gives companies a competitive advantage.
To succeed in AI, make sure your company has a clear plan for hiring (and retaining) the best available technology talent. Alternatively, partner with the right external team that can help you bootstrap quickly. The one thing you can’t do is settle for average or mediocre digital talent.
#5: Use strong infrastructure foundations
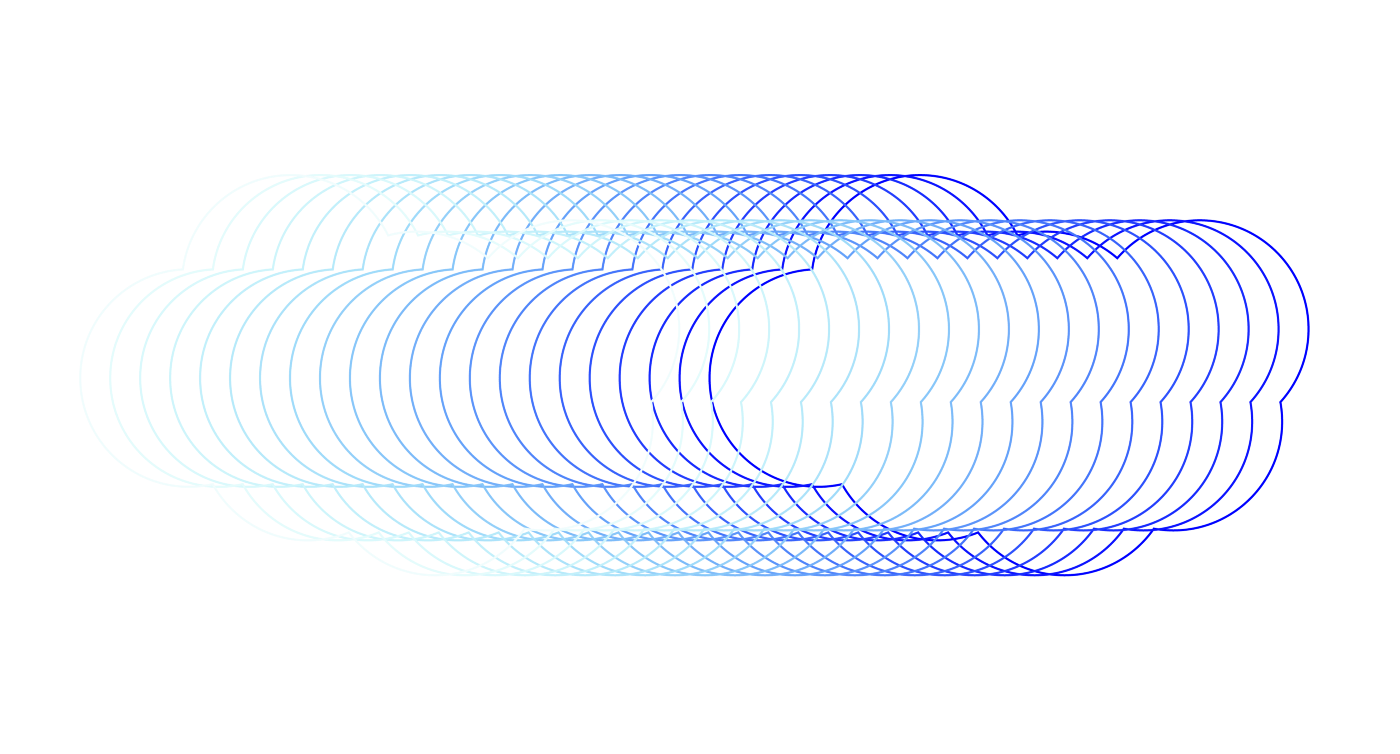
To deliver an effective AI solutions, you need an underlying data infrastructure that has sufficient storage capacity (to hold all your datasets) and sufficient computational power (to quickly process all needed calculations).
But there’s one more ingredient: your data infrastructure needs to be flexible — easy to manage and administer. Cloud services give you the ability to scale resources up or down as needed, often with a few clicks. In contrast, relying on on-premise infrastructure tends to make it harder for companies to make configuration changes at the pace that the business requires.
At Unit8 SA, we’ve found that flexible cloud infrastructure delivered by the public cloud is a core requirement for companies that want to be able to implement a sustainable advanced analytics solution.
To ensure that your data team can focus the majority of its time on value generation (and not infrastructure management), we strongly recommend a cloud-based data infrastructure approach.
#6: Don’t forget the business translators
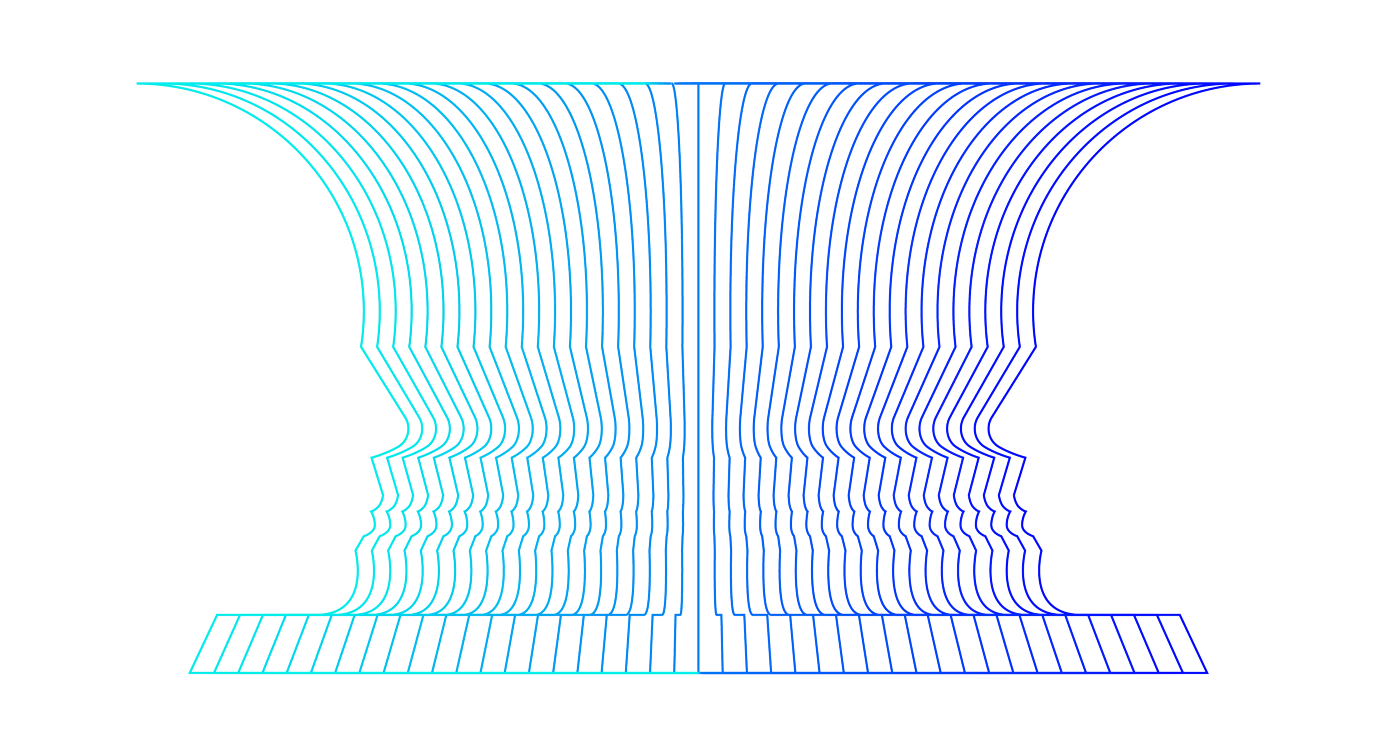
Successful analytics efforts require teams that have both data and business expertise. Without business context, data scientists and software engineers can’t be sure they are delivering the right outcome. Without technical expertise, business people can’t use technology to leverage their existing efforts.
Data scientists and software engineers often speak a language that might not be familiar to business leaders — that of machine learning models, programming languages, and AI.
The reverse is often true as well: business leaders might not know how to translate their objectives into discrete data problems.
To keep everyone on the same page and ensure successful implementation, look for analytics teams that have business-to-technology translators, or people who can keep projects on track by making sure that executives and data teams are working towards the same goals.
These translators don’t always have the same job title or background, but they are experts at scoping what is and isn’t feasible, bridging the gap between business and technology, defining business metrics that ensure accountability, and communicating progress in a way that keeps projects on budget and on time.
Conclusion
AI and analytics projects at enterprise-scale are never simple — every project we’ve worked on has brought its own unique challenges and considerations. At the same time, the principles I’ve outlined here held true at every successful Unit8 deployment.
As you begin to plan, implement, and measure your own AI or analytics program, I strongly encourage you to incorporate these principles into your plan. By adopting these best practices, you can avoid common AI pitfalls and establish a foundation for broader digital transformation at your company.
Interested in learning more? See Marcin giving a webinar on the topic
Are you trying to find the most promising data & AI use case? Watch our Unit8’s AI practice lead deliver a webinar on a methodology we use to help our customers select the right AI project
Also reach out to us at info@unit8.com — we’d love to hear from you.