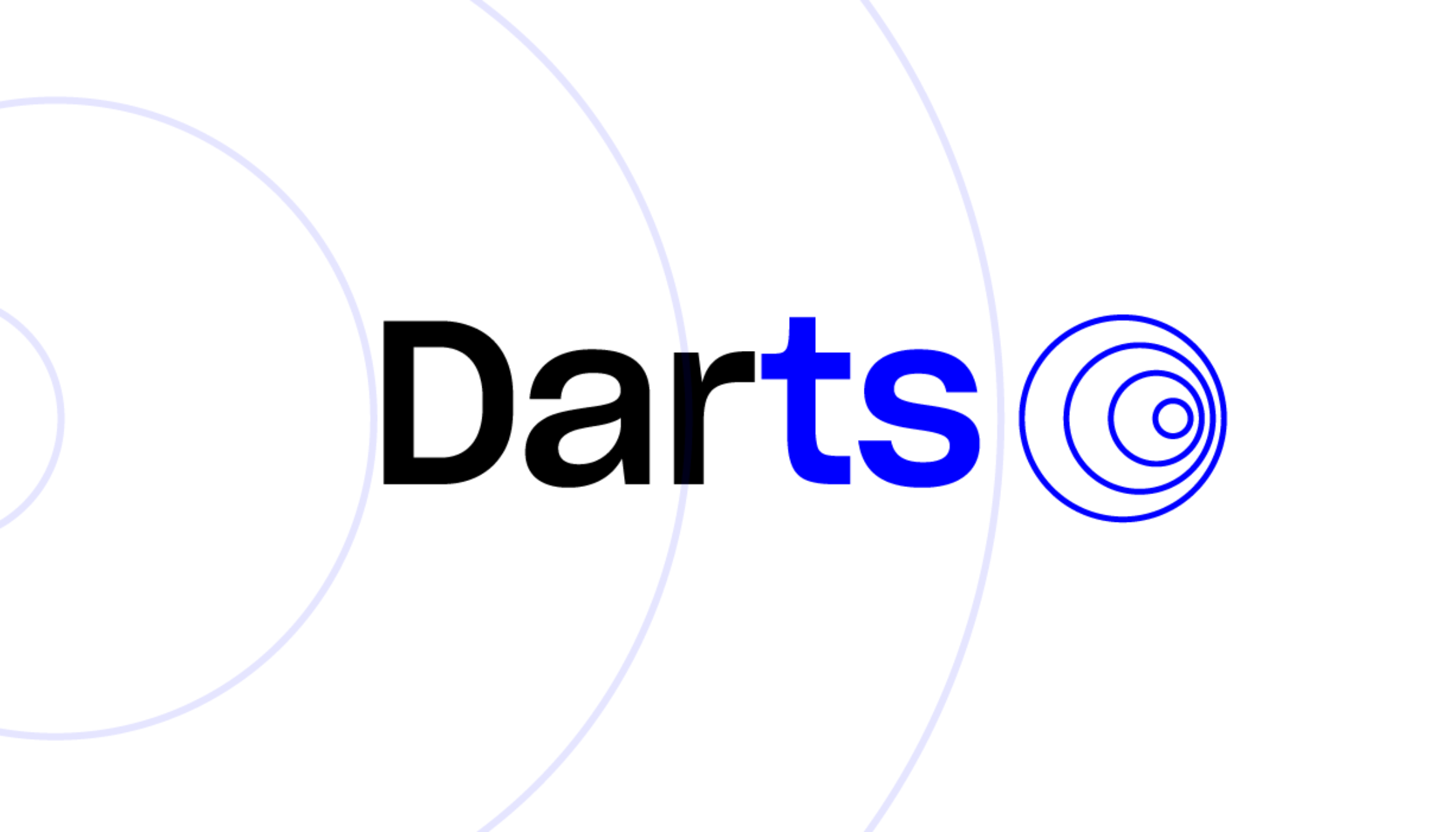
- Jul 25, 2024
- 8 minutes
-
Dennis Bader
Introduction to Forecasting
Forecasting is indispensable in today’s data-driven world. It empowers businesses to make informed decisions by predicting the future of time series based on historical data. From simple prediction tasks like daily sunrise times to more complex ones like quarter-hourly electricity demand, the applications of forecasting are vast. In this article, we highlight the power and impact of leveraging forecasting in different sectors like energy and retail. By understanding its nuances and adopting best practices, organizations can gain invaluable insights to optimize operations and enhance strategic planning.
Factors Influencing Predictability
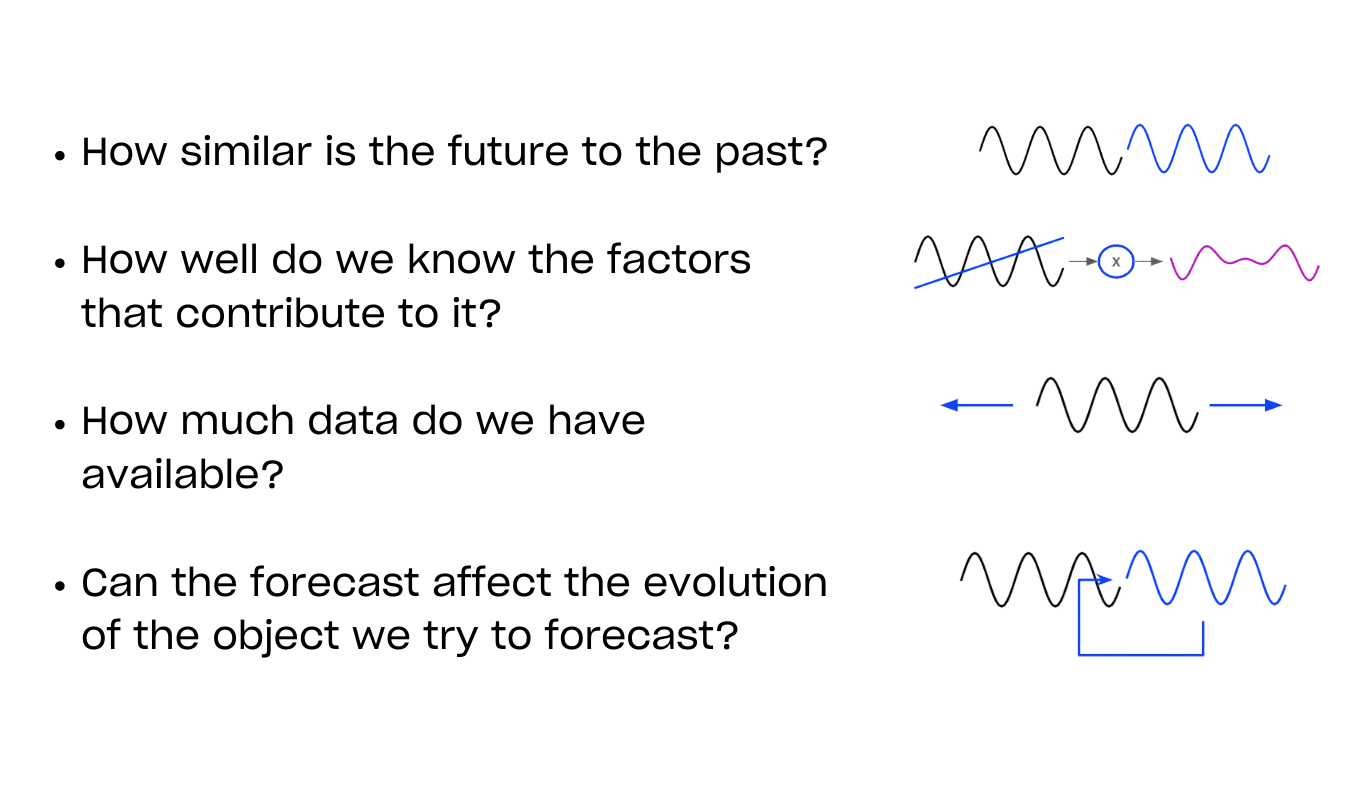
Source: Hyndman, R.J., & Athanasopoulos, G. (2021) Forecasting: principles and practice, 3rd edition, OTexts: Melbourne, Australia. OTexts.com/fpp3. Accessed on 19 May 2024
The predictability of a time series depends on several factors, including the similarity between its past and future, the granularity and quality of available data, and how the forecast itself impacts the subject being predicted. For instance, seasonal and cyclical behaviours, such as daily energy consumption, are easier to forecast than unexpected, irregular changes. The better aligned the historical and future patterns are, the more accurate the forecasts will be. Additionally, incorporating relevant external data can further enhance these predictions, reduce uncertainty and enable more precise outcomes.
Best Practices for Forecasting
Effective forecasting demands a systematic approach. Best practices involve a clear definition of the forecasting task, selecting pertinent data, and choosing appropriate models. Developing a robust forecasting pipeline is crucial and comprises several steps:
- Data Processing: Cleaning and preparing the data to ensure it is suitable for analysis.
- Feature Engineering: Creating relevant input features that capture essential patterns and relationships within the data.
- Model Selection: Choosing the right statistical or machine learning models suitable for the specific forecasting challenge.
- Evaluation: Assessing model performance through various accuracy and business-related metrics.
- Monitoring: Continuously tracking model performance to ensure its effectiveness over time, making adjustments as necessary.
Introduction to Darts
Darts, Unit8’s open-source Python library, simplifies the complex task of time series forecasting and anomaly detection. Featuring a user-friendly API, Darts supports a wide range of models, from classical statistical techniques to modern machine learning and deep learning approaches. The library offers a variety of tools for data manipulation, model training, and evaluation, making the forecasting process more efficient. By facilitating easy model comparison and providing comprehensive evaluation capabilities, Darts boosts prediction accuracy and timely anomaly detection, helping businesses make proactive decisions.
Forecasting Applications in the Real World
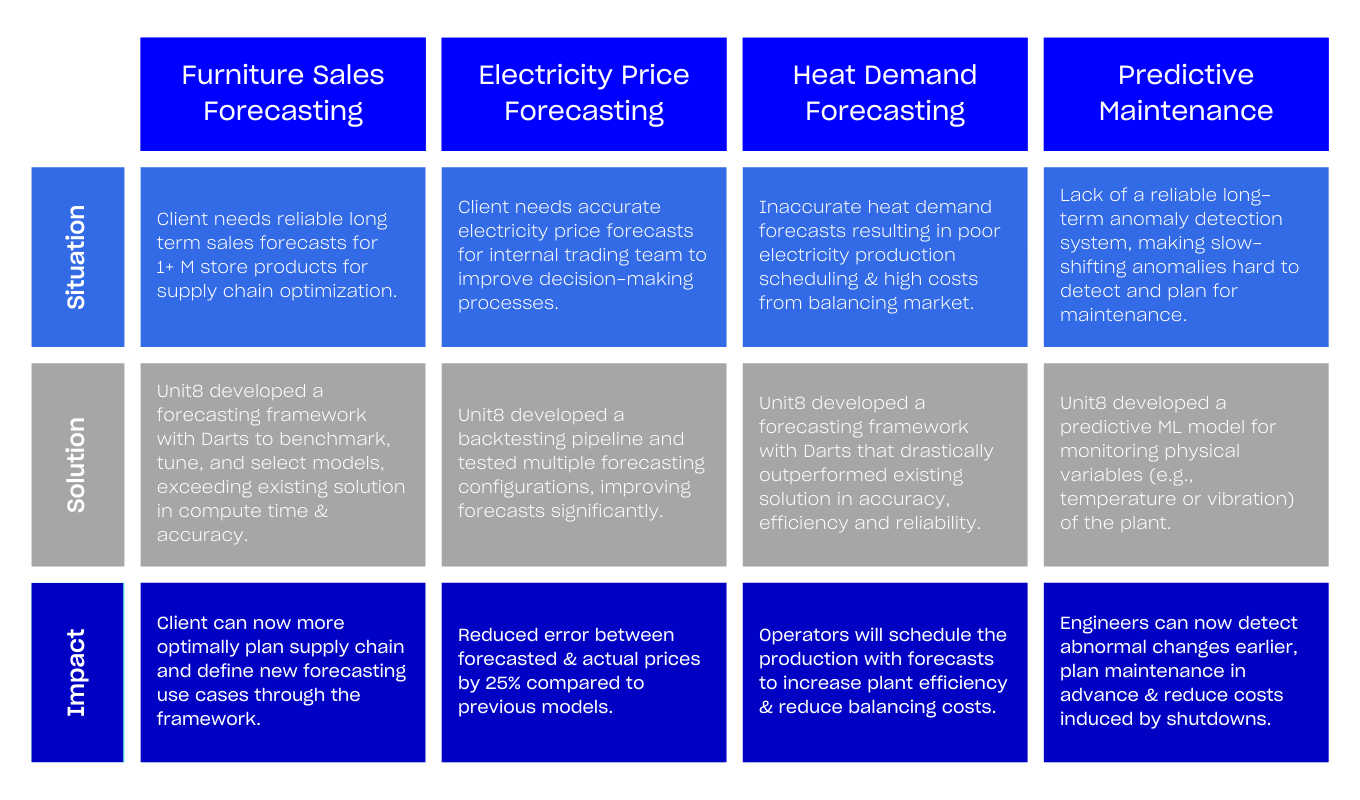
Forecasting has practical implications across multiple industries. Consider the energy sector: precise energy demand forecasting aids in resource allocation and enhances grid reliability. Supply chain optimization leverages forecasting to balance inventory levels and mitigate stock-out risks. In predictive maintenance, forecasting models anticipate equipment failures, allowing proactive maintenance, thereby reducing downtime and costs. Numerous projects demonstrate the effectiveness of forecasting frameworks like Darts in improving planning, efficiency, and accuracy, significantly impacting various industries’ operations and strategic approaches.
Conclusion
In an era where data is pivotal, robust forecasting provides a competitive edge. It enables businesses to optimize resources, reduce costs, and enhance efficiency. Leveraging best practices and powerful tools like the Darts transforms raw data into actionable insights to drive informed decision-making across various sectors.